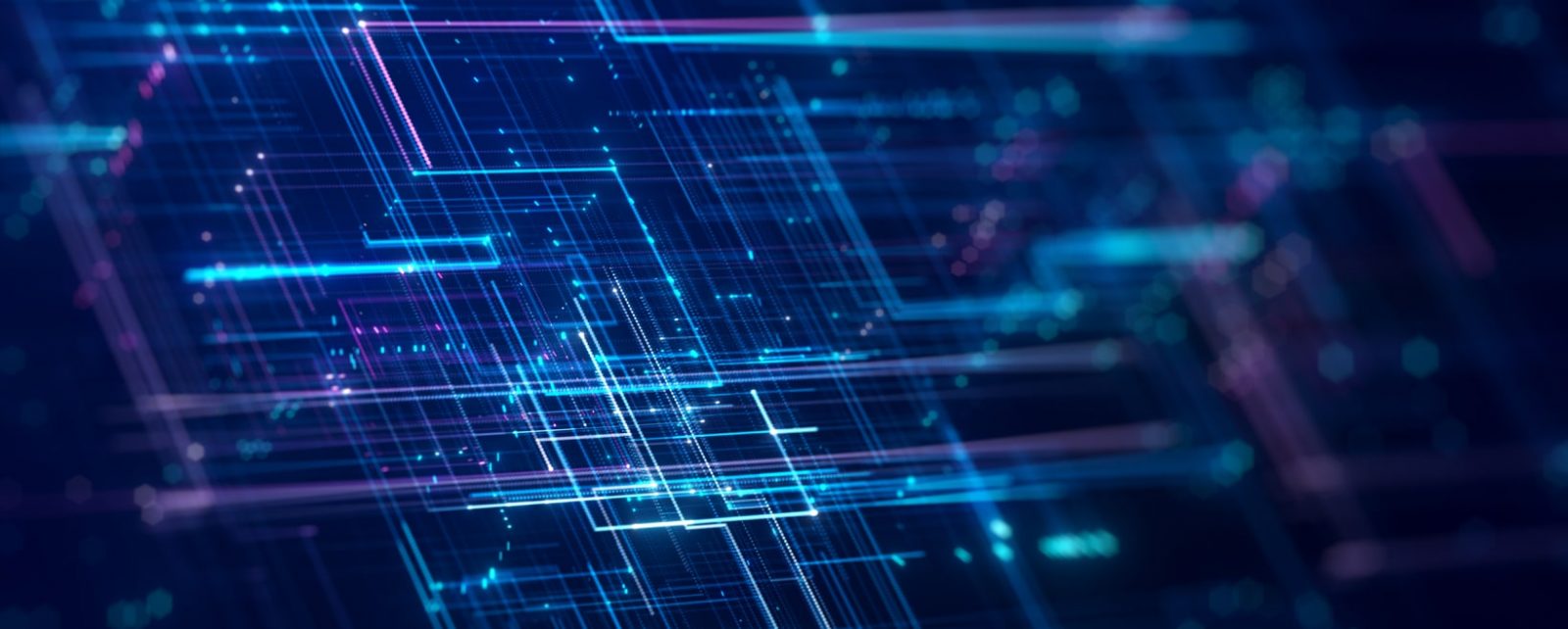
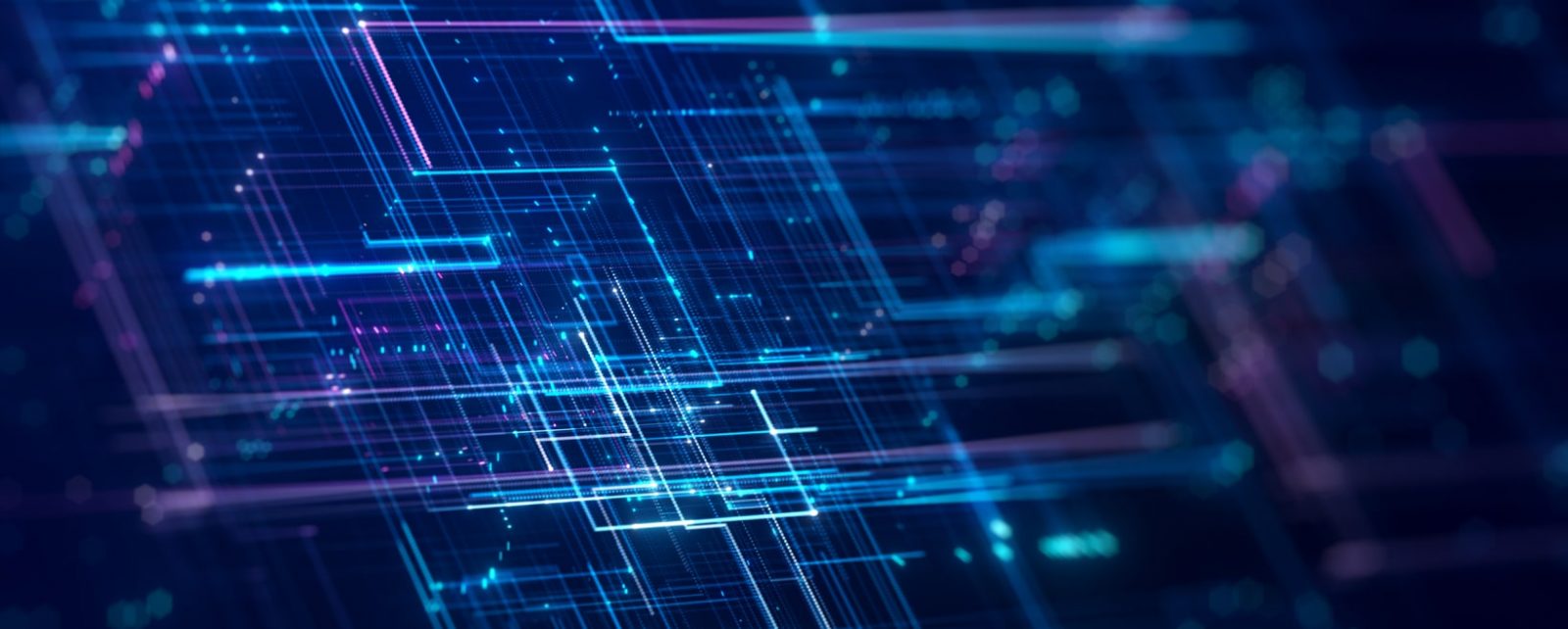
Revenue Cycle Management (RCM) is an essential function within the healthcare industry, involving all administrative and clinical tasks necessary for capturing, managing, and collecting revenue from patient services. The financial stability of healthcare organizations is largely contingent upon the effectiveness of their RCM processes. Traditionally, these processes have relied on manual labor, which often results in inefficiencies, errors, and financial setbacks.
The introduction of Artificial Intelligence (AI) and Machine Learning (ML) has profoundly altered this dynamic. These technologies are transforming how healthcare providers oversee their revenue cycles, presenting new opportunities for automation, increased accuracy, and improved decision-making. This article examines the impact of AI and ML on RCM, highlighting current uses, emerging innovations, and anticipated trends.
Current Applications of AI and ML in RCM
AI and ML are already having a notable influence on RCM by streamlining routine tasks, enhancing precision, and delivering actionable insights.
- Automated Billing and Coding: Billing and coding are fundamental to RCM but are often susceptible to errors due to the intricate nature of healthcare coding and the manual processes involved. AI systems can evaluate medical records and assign the appropriate codes automatically, thus minimizing human error and expediting billing. Machine learning algorithms refine their accuracy by learning from historical coding behaviors, ensuring adherence to regulatory requirements.
- Claims Management: Claim denials represent a significant challenge in RCM, causing delays or loss of revenue. AI tools can scrutinize past claims data to identify trends and forecast the likelihood of denials. These systems provide immediate feedback to avert errors prior to claim submission and can also automate the appeals process by generating precise appeal letters based on the reasons for denial and relevant documentation.
- Patient Eligibility Verification: Confirming patients’ eligibility for services and insurance coverage is crucial to prevent billing complications. AI can automate this verification by accessing various databases to validate insurance coverage and patient eligibility in real-time, thereby alleviating administrative burdens and reducing the chance of payment delays.
- Revenue Forecasting: AI and ML algorithms can sift through large data sets to forecast future revenue trends. By analyzing historical billing data, patient volume, and seasonal variations, these tools equip healthcare organizations with reliable revenue predictions, facilitating better financial planning and resource allocation.
- Fraud Detection: AI models are increasingly utilized to identify fraudulent billing practices. These systems can analyze extensive data sets to spot anomalies and unusual patterns, such as duplicate claims or bill services that were not rendered. Early fraud detection aids healthcare providers in mitigating financial risks and ensuring compliance with legal standards.
Emerging Developments in AI and ML for RCM
As AI and ML technologies progress, new applications are surfacing that promise to enhance the efficiency and effectiveness of RCM.
- Natural Language Processing (NLP): NLP, a branch of AI, allows machines to interpret and process human language. Within RCM, NLP can extract pertinent information from unstructured data sources like clinical notes, emails, and patient feedback, thereby improving billing accuracy, patient communication, and administrative efficiency.
- Predictive Analytics for Patient Payments: With the rise of high-deductible health plans, managing patient payments is becoming increasingly challenging. ML-powered predictive analytics can evaluate a patient’s financial history, demographics, and payment behavior to forecast their likelihood of timely payment. This insight enables healthcare providers to implement targeted payment plans and enhance collections.
- Robotic Process Automation (RPA) with AI: RPA employs software robots to automate repetitive tasks. When integrated with AI, RPA becomes more intelligent, capable of managing intricate workflows in RCM. For instance, AI-enhanced RPA can oversee comprehensive processes such as patient registration, claims submission, and payment posting, significantly alleviating the administrative load on healthcare personnel.
- AI-Driven Patient Engagement: Enhancing patient engagement is vital for improving collections and overall satisfaction. AI-powered chatbots and virtual assistants are being utilized to aid patients with billing inquiries, payment choices, and appointment scheduling. These tools offer personalized support around the clock, enhancing the patient experience while decreasing administrative demands.
- Real-Time Data Analytics: Emerging AI systems provide real-time analytics capabilities, enabling healthcare providers to track key performance indicators (KPIs) like claims denial rates, collection efficiency, and patient payment trends. Real-time insights facilitate prompt decision-making and proactive adjustments to RCM strategies.
Future Trends in AI and ML for RCM
The future of RCM is expected to undergo further transformation as AI and ML technologies continue to evolve. Several trends are anticipated to shape the future of RCM.
- Integration with Blockchain Technology: Merging AI with blockchain could revolutionize RCM by enhancing data security, transparency, and interoperability. Blockchain’s decentralized ledger technology ensures that patient and financial data remain secure and unaltered. When combined with AI, blockchain can facilitate data sharing among stakeholders, reducing inefficiencies and enhancing trust.
- Personalized Revenue Cycle Strategies: Future AI systems are likely to develop tailored RCM strategies for individual healthcare organizations, considering factors such as patient demographics, local regulations, and organizational objectives to optimize all facets of the revenue cycle.
- Advanced Fraud Prevention: Future AI models are expected to be even more adept at identifying and preventing fraud. Utilizing deep learning techniques, these models will analyze complex datasets to reveal subtle fraudulent patterns. Additionally, they will swiftly adapt to new fraud tactics, providing a strong defense against evolving threats.
- Augmented Decision-Making: AI will increasingly function as a decision-support tool, offering healthcare administrators actionable insights to enhance RCM processes. For example, AI could propose optimal pricing strategies, identify cost-saving opportunities, and recommend methods to bolster patient payment compliance, thereby enabling data-driven decision-making throughout the organization.
- End-to-End Automation: The ultimate objective of AI and ML in RCM is to achieve comprehensive automation, integrating all RCM components from patient registration to final payment. Advanced AI systems will autonomously manage complex processes, requiring minimal human intervention. This level of automation aims to maximize efficiency, lower costs, and ensure precise and timely revenue collection.
Challenges and Considerations
While the advantages of AI and ML in RCM are considerable, several challenges and considerations must be addressed.
- Data Privacy and Security: Given the sensitivity of healthcare data, the implementation of AI in RCM raises significant concerns regarding data privacy and security. Organizations must ensure compliance with regulations such as HIPAA and adopt robust cybersecurity measures to safeguard patient information.
- Implementation Costs: The initial costs associated with AI and ML technologies can be considerable, presenting a barrier for smaller healthcare providers. Nevertheless, the long-term savings and efficiency benefits often justify the initial investment.
- Workforce Adaptation: The integration of AI into RCM necessitates a shift in workforce skills. Employees must be trained to interact with AI systems and interpret their outputs. Additionally, concerns regarding job displacement need to be addressed through reskilling and upskilling initiatives.
- Regulatory Compliance: AI-driven RCM systems must adhere to the constantly evolving landscape of healthcare regulations. Ensuring compliance is crucial to avoid legal and financial penalties.
AI and ML are reshaping Revenue Cycle Management by automating processes, enhancing precision, and providing valuable insights. Current applications like automated billing, claims management, and fraud detection are already yielding considerable benefits. Emerging technologies such as NLP, predictive analytics, and AI-driven patient engagement hold the potential to further improve RCM efficiency.
Looking forward, trends such as blockchain integration, advanced fraud prevention, and end-to-end automation will redefine the RCM landscape. Despite inherent challenges, the incorporation of AI and ML in RCM presents significant opportunities for optimizing financial performance and enhancing patient outcomes in the healthcare sector.
Author: Ayana Feyisa, Technology and Analytics Services, Healthrise.
Supporting References
-
- Healthcare IT News. (2024, August 14). *AI in RCM: Healthcare execs optimistic but skeptical*.
- Inovalon. (2024, November 8). *Exploring AI’s role in revenue cycle management*.
- Kovalenko, P. (2024, September 6). Claim denial prediction: Harnessing AI for healthcare revenue cycle management. Forbes.
- Medical Economics. (2024, November 18). AI’s role in reshaping revenue cycle management.
Discover how our technology can help you reach your goals, contact us today!